《呂氏春秋‧慎行論》
察傳
夫得言不可以不察,數傳而白為黑,黑為白。故狗似玃,玃似母猴 ,母猴似人,人之與狗則遠矣。此愚者之所以大過也。聞而審則為福矣,聞而不審,不若無聞矣。齊桓公聞管子於鮑叔,楚莊聞孫叔敖於沈尹筮,審之也,故國霸諸侯也。吳王聞越王句踐於太宰嚭,智伯聞趙襄子於張武,不審也,故國亡身死也。
凡聞言必熟論,其於人必驗之以理。魯哀公問於孔子曰:「樂正夔一足,信乎?」孔子曰:「昔者舜欲以樂傳教於天下,乃令重黎舉夔於草莽之中而進之,舜以為樂正。夔於是正六律,和五聲,以通八風,而天下大服。重黎又欲益求人,舜曰:『夫樂,天地之精也 ,得失之節也,故唯聖人為能和。樂之本也。夔能和之,以平天下 。若夔者一而足矣。』故曰夔一足,非一足也。」宋之丁氏,家無井而出溉汲,常一人居外。及其家穿井,告人曰:「吾穿井得一人 。」有聞而傳之者曰:「丁氏穿井得一人。」國人道之,聞之於宋君,宋君令人問之於丁氏,丁氏對曰:「得一人之使,非得一人於井中也。」求能之若此,不若無聞也。
子夏之晉,過衛,有讀史記者曰:「晉師三豕涉河。」子夏曰:「非也,是己亥也。夫『己』與『三』相近,『豕』與『亥』相似 。」至於晉而問之,則曰「晉師己亥涉河」也。辭多類非而是,多類是而非。是非之經,不可不分,此聖人之所慎也。然則何以慎?緣物之情及人之情以為所聞則得之矣。
古早的中國喜用『類比』來論事說理,難道這就是『科學』不興的原因嗎?李約瑟在其大著《中國的科學與文明》試圖解決這個今稱『李約瑟難題』之大哉問!終究還是百家爭鳴也?若是比喻的說︰一個孤立隔絕系統之演化,常因內部機制的折衝協調,周遭環境之影響相對的小很多。因此秦之『大一統』,歷代的『戰亂』頻起,能不達於『社會』之『平衡』的耶??如此『主流價值』亦是已然確立成為『文化內涵』的吧!!所以『天不變』、『道不變』,人亦『不變』乎!!??雖然李約瑟曾經明示『類比』── 關聯式 corelative thinking 思考 ── 難以建立完整的『邏輯體系』, 或是『科學』不興的理由耶??!!如果『自然事物』之『邏輯推理』能形成系統『大樹』,那麼『類比關聯』將創造體系『森林』矣,豈可不『慎察』也。
類比(英語:Analogy,源自古希臘語:ἀναλογία,analogia,意為等比例的),或類推,是一種認知過程,將某個特定事物所附帶的訊息轉移到其他特定事物之上。類比通過比較兩件事情,清楚揭示二者之間的相似點,並將已知事物的特點,推衍到未知事物中,但兩者不一定有實質上的同源性,其類比也不見得「合理」。在記憶、溝通與問題解決等過程中扮演重要角色;於不同學科中也有各自的定義。
舉例而言,原子中的原子核以及由電子組成的軌域,可類比成太陽系中行星環繞太陽的樣子。除此之外,修辭學中的譬喻法有時也是一種類比,例如將月亮比喻成銀幣。生物學中因趨同演化而形成的的同功或同型解剖構造,例如哺乳類、爬行類與鳥類的翅膀也是類似概念。
───
Analogy
Analogy (from Greek ἀναλογία, analogia, “proportion”[1][2]) is a cognitive process of transferring information or meaning from a particular subject (the analogue or source) to another (the target), or a linguistic expression corresponding to such a process. In a narrower sense, analogy is an inference or an argument from one particular to another particular, as opposed to deduction, induction, and abduction, where at least one of the premises or the conclusion is general. The word analogy can also refer to the relation between the source and the target themselves, which is often, though not necessarily, a similarity, as in the biological notion of analogy.
Analogy plays a significant role in problem solving such as, decision making, perception, memory, creativity, emotion, explanation, and communication. It lies behind basic tasks such as the identification of places, objects and people, for example, in face perception and facial recognition systems. It has been argued that analogy is “the core of cognition”.[3] Specific analogical language comprises exemplification, comparisons, metaphors, similes, allegories, and parables, but not metonymy. Phrases like and so on, and the like, as if, and the very word like also rely on an analogical understanding by the receiver of a message including them. Analogy is important not only in ordinary language and common sense (where proverbs and idioms give many examples of its application) but also in science, philosophy, and the humanities. The concepts of association, comparison, correspondence, mathematical and morphological homology, homomorphism, iconicity, isomorphism, metaphor, resemblance, and similarity are closely related to analogy. In cognitive linguistics, the notion of conceptual metaphor may be equivalent to that of analogy.
Analogy has been studied and discussed since classical antiquity by philosophers, scientists, and lawyers. The last few decades have shown a renewed interest in analogy, most notably in cognitive science.
Rutherford’s model of the atom (modified by Niels Bohr) made an analogy between the atom and the solar system.
───
將如何了解 Michael Nielsen 先生所言之『雜訊』的呢?
Noise is a variety of sound, usually meaning any unwanted sound.
Noise may also refer to:
Random or unwanted signals
假使藉著『取樣原理』將 MNIST 『手寫阿拉伯數字』看成『函式』
Whittaker–Shannon interpolation formula
The Whittaker–Shannon interpolation formula or sinc interpolation is a method to construct a continuous-time bandlimited function from a sequence of real numbers. The formula dates back to the works of E. Borel in 1898, and E. T. Whittaker in 1915, and was cited from works of J. M. Whittaker in 1935, and in the formulation of the Nyquist–Shannon sampling theorem by Claude Shannon in 1949. It is also commonly called Shannon’s interpolation formula and Whittaker’s interpolation formula. E. T. Whittaker, who published it in 1915, called it the Cardinal series.
Definition
Given a sequence of real numbers, x[n], the continuous function
(where “sinc” denotes the normalized sinc function) has a Fourier transform, X(f), whose non-zero values are confined to the region |f| ≤ 1/(2T). When parameter T has units of seconds, the bandlimit, 1/(2T), has units of cycles/sec (hertz). When the x[n] sequence represents time samples, at interval T, of a continuous function, the quantity fs = 1/T is known as the sample rate, and fs/2 is the corresponding Nyquist frequency. When the sampled function has a bandlimit, B, less than the Nyquist frequency, x(t) is a perfect reconstruction of the original function. (See Sampling theorem.) Otherwise, the frequency components above the Nyquist frequency “fold” into the sub-Nyquist region of X(f), resulting in distortion. (See Aliasing.)
Fourier transform of a bandlimited function.
─── 摘自《勇闖新世界︰ W!o《卡夫卡村》變形祭︰品味科學‧教具教材‧【專題】 PD‧箱子世界‧取樣》
自然可藉著
變分法
變分法是處理泛函的數學領域,和處理函數的普通微積分相對。譬如,這樣的泛函可以通過未知函數的積分和它的導數來構造。變分法最終尋求的是極值函數:它們使得泛函取得極大或極小值。有些曲線上的經典問題採用這種形式表達:一個例子是最速降線,在重力作用下一個粒子沿著該路徑可以在最短時間從點A到達不直接在它底下的一點B。在所有從A到B的曲線中必須極小化代表下降時間的表達式。
變分法的關鍵定理是歐拉-拉格朗日方程。它對應於泛函的臨界點。在尋找函數的極大和極小值時,在一個解附近的微小變化的分析給出一階的一個近似。它不能分辨是找到了最大值或者最小值(或者都不是)。
變分法在理論物理中非常重要:在拉格朗日力學中,以及在最小作用量原理在量子力學的應用中。變分法提供了有限元方法的數學基礎,它是求解邊界值問題的強力工具。它們也在材料學中研究材料平衡中大量使用。而在純數學中的例子有,黎曼在調和函數中使用狄利克雷原理。
同樣的材料可以出現在不同的標題中,例如希爾伯特空間技術,莫爾斯理論,或者辛幾何。變分一詞用於所有極值泛函問題。微分幾何中的測地線的研究是很顯然的變分性質的領域。極小曲面(肥皂泡)上也有很多研究工作,稱為普拉托問題。
───
用『任意鄰近函式』 的概念
Euler–Lagrange equation
Finding the extrema of functionals is similar to finding the maxima and minima of functions. The maxima and minima of a function may be located by finding the points where its derivative vanishes (i.e., is equal to zero). The extrema of functionals may be obtained by finding functions where the functional derivative is equal to zero. This leads to solving the associated Euler–Lagrange equation.[Note 3]
Consider the functional
where
- x1, x2 are constants,
- y (x) is twice continuously differentiable,
- y ′(x) = dy / dx ,
- L(x, y (x), y ′(x)) is twice continuously differentiable with respect to its arguments x, y, y ′.
If the functional J[y ] attains a local minimum at f , and η(x) is an arbitrary function that has at least one derivative and vanishes at the endpoints x1 and x2 , then for any number ε close to 0,
The term εη is called the variation of the function f and is denoted by δf .[11]
Substituting f + εη for y in the functional J[ y ] , the result is a function of ε,
Since the functional J[ y ] has a minimum for y = f , the function Φ(ε) has a minimum at ε = 0 and thus,[Note 4]
Taking the total derivative of L[x, y, y ′] , where y = f + ε η and y ′ = f ′ + ε η′ are functions of ε but x is not,
and since dy /dε = η and dy ′/dε = η’ ,
.
Therefore,
where L[x, y, y ′] → L[x, f, f ′] when ε = 0 and we have used integration by parts. The last term vanishes because η = 0 at x1 and x2 by definition. Also, as previously mentioned the left side of the equation is zero so that
According to the fundamental lemma of calculus of variations, the part of the integrand in parentheses is zero, i.e.
which is called the Euler–Lagrange equation. The left hand side of this equation is called the functional derivative of J[f] and is denoted δJ/δf(x) .
In general this gives a second-order ordinary differential equation which can be solved to obtain the extremal function f(x) . The Euler–Lagrange equation is a necessary, but not sufficient, condition for an extremum J[f]. A sufficient condition for a minimum is given in the section Variations and sufficient condition for a minimum.
───
來考察所有『辨識』為某數的『手寫阿拉伯數字』之『相似性』
也可探究因『權重』 weight 之『隨機賦值』或『圖像雜訊』所可能引發的現象與解決之發想乎?
Total variation denoising
In signal processing, Total variation denoising, also known as total variation regularization is a process, most often used in digital image processing, that has applications in noise removal. It is based on the principle that signals with excessive and possibly spurious detail have high total variation, that is, the integral of the absolute gradient of the signal is high. According to this principle, reducing the total variation of the signal subject to it being a close match to the original signal, removes unwanted detail whilst preserving important details such as edges. The concept was pioneered by Rudin et al. in 1992.[1]
This noise removal technique has advantages over simple techniques such as linear smoothing or median filtering which reduce noise but at the same time smooth away edges to a greater or lesser degree. By contrast, total variation denoising is remarkably effective at simultaneously preserving edges whilst smoothing away noise in flat regions, even at low signal-to-noise ratios.[2]
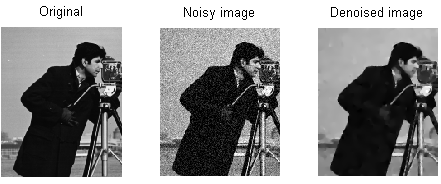
Mathematical exposition for 1D digital signals
For a digital signal , we can, for example, define the total variation as:
Given an input signal , the goal of total variation denoising is to find an approximation, call it
, that has smaller total variation than
but is “close” to
. One measure of closeness is the sum of square errors:
So the total variation denoising problem amounts to minimizing the following discrete functional over the signal :
By differentiating this functional with respect to , we can derive a corresponding Euler–Lagrange equation, that can be numerically integrated with the original signal
as initial condition. This was the original approach.[1] Alternatively, since this is a convex functional, techniques from convex optimization can be used to minimize it and find the solution
.[3]
Application of 1D total variation denoising to a signal obtained from a single-molecule experiment.[3] Gray is the original signal, black is the denoised signal.
Regularization properties
The regularization parameter plays a critical role in the denoising process. When
, there is no denoising and the result is identical to the input signal. As
, however, the total variation term plays an increasingly strong role, which forces the result to have smaller total variation, at the expense of being less like the input (noisy) signal. Thus, the choice of regularization parameter is critical to achieving just the right amount of noise removal.
───
植種大樹,走入森林,方知
縱使宇宙萬有同源,萬象表現實在是錯綜複雜耶!!方了
世間書籍雖然汗牛充棟,原創概念往往卻沒有幾個??