《夏日》北宋‧張耒
長夏江村風日清,簷牙燕雀已生成。
蝶衣曬粉花枝午,蛛網添絲屋角晴。
落落疏簾邀月影,嘈嘈虛枕納溪聲。
久斑兩鬢如霜雪,直欲樵漁過此生。
《輸麥行》
場頭雨乾場地白,老穉相呼打新麥。
半歸倉廩半輸官,免教縣吏相催迫。
羊頭車子毛巾囊,淺泥易涉登前岡。
倉頭買券槐陰凉,清嚴官吏兩平量。
出倉掉臂呼同伴,旗亭酒美單衣換。
半醉扶車歸路凉,月出到家妻具飯。
一年從此皆閒日,風雨閉門公事畢。
射狐罝兔歲蹉跎,百壺社酒相經過。
(自注:此篇效張文昌,而語差繁)
聖嬰正反交替之際,未入暑,熱先至!怎得清涼乎?哪曉 M♪o 竟能『讀得一片清涼』
夏夜歎‧杜甫
永日不可暮,炎蒸毒我腸。
安得萬里風,飄飖吹我裳。
昊天出華月,茂林延疏光。
仲夏苦夜短,開軒納微涼。
虛明見纖毫,羽蟲亦飛颺。
物情無巨細,自適固其常。
念彼荷戈士,窮年守邊疆。
何由一洗濯,執熱互相望。
竟夕擊刁鬥,喧聲連萬方。
青紫雖被體,不如早還鄉。
北城悲笳發,鸛鶴號且翔。
況複煩促倦,激烈思時康。
未濟:亨,小狐汔濟,濡其尾,無攸利。
彖曰:未濟,亨﹔柔得中也。 小狐汔濟,未出中也。 濡其尾,無攸利﹔不續終也。 雖不當位,剛柔應也。
象曰:火在水上,未濟﹔君子以慎辨物居方 。
,既無攸利,小狐何事濡其尾,來汔濟??問前途,恐有大水大火交替!!
無課自習。 ☿☹
派︰暑熱難讀文章,炎蒸令人神喪。也不知道誰講︰『心靜自然涼』。莫言永日天光長,遁入自好將己忘。讀得一片清涼︰
《木蘭辭》‧佚名
唧唧復唧唧,木蘭當戶織。不聞機杼聲,惟聞女嘆息。
問女何所思?問女何所憶?女亦無所思,女亦無所憶。
昨夜見軍帖,可汗大點兵。軍書十二卷,卷卷有爺名。
阿爺無大兒,木蘭無長兄。願為市鞍馬,從此替爺征。
東市買駿馬,西市買鞍韉,南市買轡頭,北市買長鞭。
朝辭爺孃去,暮宿黃河邊,
不聞爺孃喚女聲,但聞黃河流水聲濺濺。
旦辭黃河去,暮宿黑山頭;
不聞爺孃喚女聲,但聞燕山胡騎聲啾啾。
萬里赴戎機,關山度若飛。朔氣傳金柝,寒光照鐵衣。
將軍百戰死,壯士十年歸。歸來見天子,天子坐明堂。
策勳十二轉,賞賜百千強。可汗問所欲 木蘭不用尚書郎,
願借明駝千里足,送兒還故鄉。
爺孃聞女來,出郭相扶將。阿姊聞妹來,當戶理紅妝。
小弟聞姊來,磨刀霍霍向豬羊。
開我東閣門,坐我西閣床。
脫我戰時袍,著我舊時裳,當窗理雲鬢,對鏡貼花黃。
出門看火伴,火伴皆驚惶:同行十二年,不知木蘭是女郎。
雄兔腳撲朔,雌兔眼迷離,兩兔傍地走,安能辨我是雄雌?
沉思『唧唧』是何聲響?故非機杼響,當真嘆息聲!逐一細點去,水聲寫濺濺, 騎聲用啾啾,皆是形聲法,象物之擬音。玄想曾經『何時』『哪地』人,嘆息聲『唧唧』??還有那『喚女聲』,…『朔氣傳金柝,寒光照鐵衣。』,忽憶
《塞下曲》‧盧綸
月黑雁飛高,單于夜遁逃。
欲將輕騎逐,大雪滿弓刀。
,此時『秋蟬』死,不該是『知了』,難不成會是『守宮』!! ☺☺ 一時哈哈大笑,領悟了『讀碼』之 技︰天地一指,萬物一
『馬』,同樣是『文』『章』。
─ 摘自《M♪o 之學習筆記本《寅》井井︰【黃土長夏】永日炎蒸》
不知 Michael Nielsen 先生大筆一揮之文,可有同樣功效的耶??
Introducing convolutional networks
In earlier chapters, we taught our neural networks to do a pretty good job recognizing images of handwritten digits:
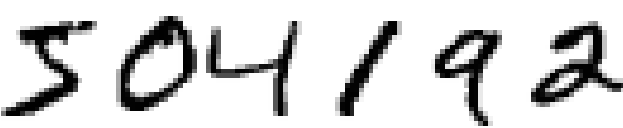
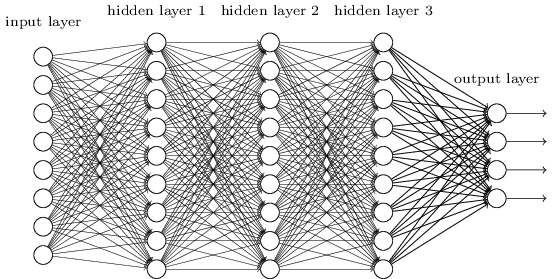
Our earlier networks work pretty well: we’ve obtained a classification accuracy better than 98 percent, using training and test data from the MNIST handwritten digit data set. But upon reflection, it’s strange to use networks with fully-connected layers to classify images. The reason is that such a network architecture does not take into account the spatial structure of the images. For instance, it treats input pixels which are far apart and close together on exactly the same footing. Such concepts of spatial structure must instead be inferred from the training data. But what if, instead of starting with a network architecture which is tabula rasa, we used an architecture which tries to take advantage of the spatial structure? In this section I describe convolutional neural networks*
*The origins of convolutional neural networks go back to the 1970s. But the seminal paper establishing the modern subject of convolutional networks was a 1998 paper, “Gradient-based learning applied to document recognition”, by Yann LeCun, Léon Bottou, Yoshua Bengio, and Patrick Haffner. LeCun has since made an interesting remark on the terminology for convolutional nets: “The [biological] neural inspiration in models like convolutional nets is very tenuous. That’s why I call them ‘convolutional nets’ not ‘convolutional neural nets’, and why we call the nodes ‘units’ and not ‘neurons’ “. Despite this remark, convolutional nets use many of the same ideas as the neural networks we’ve studied up to now: ideas such as backpropagation, gradient descent, regularization, non-linear activation functions, and so on. And so we will follow common practice, and consider them a type of neural network. I will use the terms “convolutional neural network” and “convolutional net(work)” interchangeably. I will also use the terms “[artificial] neuron” and “unit” interchangeably.
. These networks use a special architecture which is particularly well-adapted to classify images. Using this architecture makes convolutional networks fast to train. This, in turns, helps us train deep, many-layer networks, which are very good at classifying images. Today, deep convolutional networks or some close variant are used in most neural networks for image recognition.
Convolutional neural networks use three basic ideas: local receptive fields, shared weights, and pooling. Let’s look at each of these ideas in turn.
Local receptive fields: In the fully-connected layers shown earlier, the inputs were depicted as a vertical line of neurons. In a convolutional net, it’ll help to think instead of the inputs as a square of neurons, whose values correspond to the
pixel intensities we’re using as inputs:
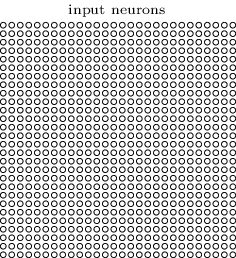
To be more precise, each neuron in the first hidden layer will be connected to a small region of the input neurons, say, for example, a region, corresponding to
input pixels. So, for a particular hidden neuron, we might have connections that look like this:
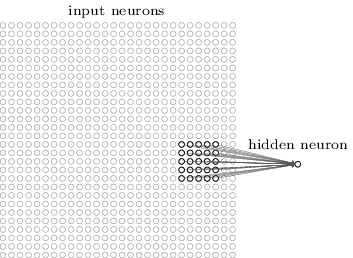
We then slide the local receptive field across the entire input image. For each local receptive field, there is a different hidden neuron in the first hidden layer. To illustrate this concretely, let’s start with a local receptive field in the top-left corner:
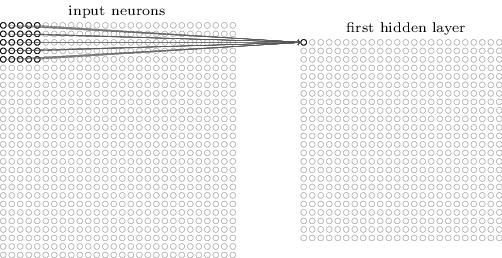
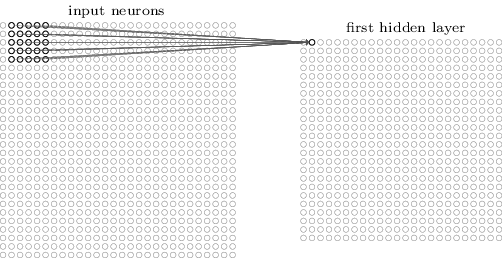





I’ve shown the local receptive field being moved by one pixel at a time. In fact, sometimes a different stride length is used. For instance, we might move the local receptive field pixels to the right (or down), in which case we’d say a stride length of
is used. In this chapter we’ll mostly stick with stride length
, but it’s worth knowing that people sometimes experiment with different stride lengths*
*As was done in earlier chapters, if we’re interested in trying different stride lengths then we can use validation data to pick out the stride length which gives the best performance. For more details, see the earlier discussion of how to choose hyper-parameters in a neural network. The same approach may also be used to choose the size of the local receptive field – there is, of course, nothing special about using a local receptive field. In general, larger local receptive fields tend to be helpful when the input images are significantly larger than the
pixel MNIST images..
Shared weights and biases: I’ve said that each hidden neuron has a bias and weights connected to its local receptive field. What I did not yet mention is that we’re going to use the same weights and bias for each of the
hidden neurons. In other words, for the
th hidden neuron, the output is:

Here, is the neural activation function – perhaps the sigmoid function we used in earlier chapters.
is the shared value for the bias.
is a
array of shared weights. And, finally, we use
to denote the input activation at position
.
This means that all the neurons in the first hidden layer detect exactly the same feature*
*I haven’t precisely defined the notion of a feature. Informally, think of the feature detected by a hidden neuron as the kind of input pattern that will cause the neuron to activate: it might be an edge in the image, for instance, or maybe some other type of shape.
, just at different locations in the input image. To see why this makes sense, suppose the weights and bias are such that the hidden neuron can pick out, say, a vertical edge in a particular local receptive field. That ability is also likely to be useful at other places in the image. And so it is useful to apply the same feature detector everywhere in the image. To put it in slightly more abstract terms, convolutional networks are well adapted to the translation invariance of images: move a picture of a cat (say) a little ways, and it’s still an image of a cat*
*In fact, for the MNIST digit classification problem we’ve been studying, the images are centered and size-normalized. So MNIST has less translation invariance than images found “in the wild”, so to speak. Still, features like edges and corners are likely to be useful across much of the input space. .
For this reason, we sometimes call the map from the input layer to the hidden layer a feature map. We call the weights defining the feature map the shared weights. And we call the bias defining the feature map in this way the shared bias. The shared weights and bias are often said to define a kernel or filter. In the literature, people sometimes use these terms in slightly different ways, and for that reason I’m not going to be more precise; rather, in a moment, we’ll look at some concrete examples.
The network structure I’ve described so far can detect just a single kind of localized feature. To do image recognition we’ll need more than one feature map. And so a complete convolutional layer consists of several different feature maps:
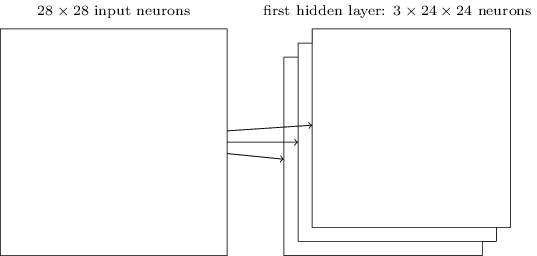



I’ve shown just feature maps, to keep the diagram above simple. However, in practice convolutional networks may use more (and perhaps many more) feature maps. One of the early convolutional networks, LeNet-5, used
feature maps, each associated to a
local receptive field, to recognize MNIST digits. So the example illustrated above is actually pretty close to LeNet-5. In the examples we develop later in the chapter we’ll use convolutional layers with
and
feature maps. Let’s take a quick peek at some of the features which are learned*
*The feature maps illustrated come from the final convolutional network we train, see here.:
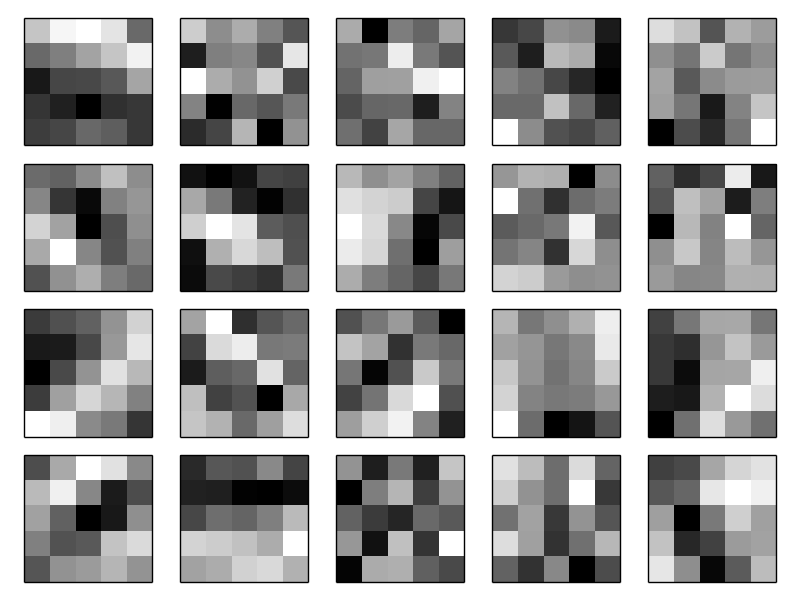




So what can we conclude from these feature maps? It’s clear there is spatial structure here beyond what we’d expect at random: many of the features have clear sub-regions of light and dark. That shows our network really is learning things related to the spatial structure. However, beyond that, it’s difficult to see what these feature detectors are learning. Certainly, we’re not learning (say) the Gabor filters which have been used in many traditional approaches to image recognition. In fact, there’s now a lot of work on better understanding the features learnt by convolutional networks. If you’re interested in following up on that work, I suggest starting with the paper Visualizing and Understanding Convolutional Networks by Matthew Zeiler and Rob Fergus (2013).
A big advantage of sharing weights and biases is that it greatly reduces the number of parameters involved in a convolutional network. For each feature map we need shared weights, plus a single shared bias. So each feature map requires
parameters. If we have
feature maps that’s a total of
parameters defining the convolutional layer. By comparison, suppose we had a fully connected first layer, with
input neurons, and a relatively modest
hidden neurons, as we used in many of the examples earlier in the book. That’s a total of
weights, plus an extra
biases, for a total of
parameters. In other words, the fully-connected layer would have more than
times as many parameters as the convolutional layer.
Of course, we can’t really do a direct comparison between the number of parameters, since the two models are different in essential ways. But, intuitively, it seems likely that the use of translation invariance by the convolutional layer will reduce the number of parameters it needs to get the same performance as the fully-connected model. That, in turn, will result in faster training for the convolutional model, and, ultimately, will help us build deep networks using convolutional layers.
Incidentally, the name convolutional comes from the fact that the operation in Equation (125) is sometimes known as a convolution. A little more precisely, people sometimes write that equation as , where
denotes the set of output activations from one feature map,
is the set of input activations, and ∗ is called a convolution operation. We’re not going to make any deep use of the mathematics of convolutions, so you don’t need to worry too much about this connection. But it’s worth at least knowing where the name comes from.
Pooling layers: In addition to the convolutional layers just described, convolutional neural networks also contain pooling layers. Pooling layers are usually used immediately after convolutional layers. What the pooling layers do is simplify the information in the output from the convolutional layer.
In detail, a pooling layer takes each feature map*
*The nomenclature is being used loosely here. In particular, I’m using “feature map” to mean not the function computed by the convolutional layer, but rather the activation of the hidden neurons output from the layer. This kind of mild abuse of nomenclature is pretty common in the research literature.
output from the convolutional layer and prepares a condensed feature map. For instance, each unit in the pooling layer may summarize a region of (say) neurons in the previous layer. As a concrete example, one common procedure for pooling is known as max-pooling. In max-pooling, a pooling unit simply outputs the maximum activation in the
input region, as illustrated in the following diagram:
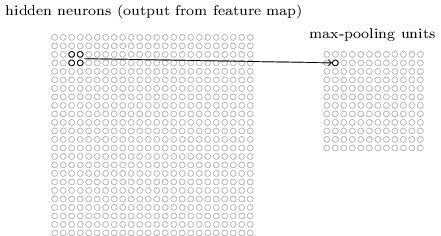


As mentioned above, the convolutional layer usually involves more than a single feature map. We apply max-pooling to each feature map separately. So if there were three feature maps, the combined convolutional and max-pooling layers would look like:
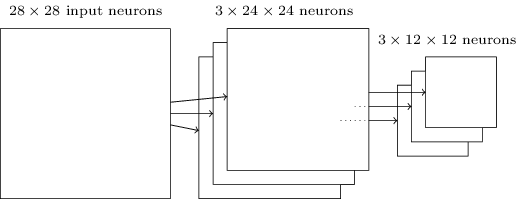
Max-pooling isn’t the only technique used for pooling. Another common approach is known as L2 pooling. Here, instead of taking the maximum activation of a region of neurons, we take the square root of the sum of the squares of the activations in the
region. While the details are different, the intuition is similar to max-pooling: L2 pooling is a way of condensing information from the convolutional layer. In practice, both techniques have been widely used. And sometimes people use other types of pooling operation. If you’re really trying to optimize performance, you may use validation data to compare several different approaches to pooling, and choose the approach which works best. But we’re not going to worry about that kind of detailed optimization.
Putting it all together: We can now put all these ideas together to form a complete convolutional neural network. It’s similar to the architecture we were just looking at, but has the addition of a layer of output neurons, corresponding to the
possible values for MNIST digits (‘0’, ‘1’, ‘2’, etc):
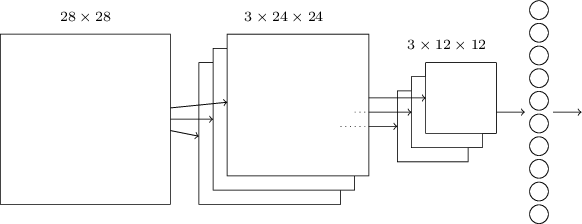







The final layer of connections in the network is a fully-connected layer. That is, this layer connects every neuron from the max-pooled layer to every one of the output neurons. This fully-connected architecture is the same as we used in earlier chapters. Note, however, that in the diagram above, I’ve used a single arrow, for simplicity, rather than showing all the connections. Of course, you can easily imagine the connections.
This convolutional architecture is quite different to the architectures used in earlier chapters. But the overall picture is similar: a network made of many simple units, whose behaviors are determined by their weights and biases. And the overall goal is still the same: to use training data to train the network’s weights and biases so that the network does a good job classifying input digits.
In particular, just as earlier in the book, we will train our network using stochastic gradient descent and backpropagation. This mostly proceeds in exactly the same way as in earlier chapters. However, we do need to make few modifications to the backpropagation procedure. The reason is that our earlier derivation of backpropagation was for networks with fully-connected layers. Fortunately, it’s straightforward to modify the derivation for convolutional and max-pooling layers. If you’d like to understand the details, then I invite you to work through the following problem. Be warned that the problem will take some time to work through, unless you’ve really internalized the earlier derivation of backpropagation (in which case it’s easy).
───
【參考資料』
‧ Fully Connected Neural Network Algorithms
‧ Convolutional Neural Networks