然而即使小汽車有『雷射指標』,處於
火星探測是指人類通過向火星發射太空探測器,對火星進行的科學探測活動。人類從1600年代開始使用望遠鏡對火星進行觀測。美國的水手4號於1964年12月28日發射升空,這是有史以來第一枚成功到達火星並發回數據的探測器。
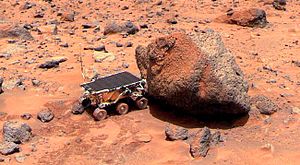
Sojourner takes its Alpha Proton X-ray Spectrometer measurement of the Yogi Rock
動畫展示各個火星探測器的登陸點
概述
火星是太陽系八大行星之一,按離太陽由近及遠的次序排列為第四顆。在太陽系八大行星之中,火星也是除了金星以外,距離地球最近的行星。大約每隔26個月就會發生一次火星沖日,地球與火星的距離在沖日期間會達到極近值,通常只有不足1億千米,而在火星發生大衝時,這個距離甚至不足6000萬千米。火星沖日意味著這時可以使用較小花費將探測器送往火星,火星探測通常也會利用此天文現象來運作。
到目前為止,已經有超過30枚探測器到達過火星,它們對火星進行了詳細的考察,並向地球發回了大量數據。同時火星探測也充滿了坎坷,大約三分之二的 探測器,特別是早期發射的探測器,都沒有能夠成功完成它們的使命。但是火星對於人類卻有一種特殊的吸引力,因為它是太陽系中最近似地球的天體之一。火星赤 道平面與公轉軌道平面的交角非常接近於地球,這使它也有類似地球的四季交替,同時,火星的自轉周期為24小時37分,這使火星上的一天幾乎和地球上的一樣 長。
環境,恐怕前途渺渺茫茫,難有用武之地也。那麼可有方法能從『動態影像』中推估『運動』呢?
Motion estimation is the process of determining motion vectors that describe the transformation from one 2D image to another; usually from adjacent frames in a video sequence. It is an ill-posed problem as the motion is in three dimensions but the images are a projection of the 3D scene onto a 2D plane. The motion vectors may relate to the whole image (global motion estimation) or specific parts, such as rectangular blocks, arbitrary shaped patches or even per pixel. The motion vectors may be represented by a translational model or many other models that can approximate the motion of a real video camera, such as rotation and translation in all three dimensions and zoom.

Motion vectors that result from a movement into the
-plane of the image, combined with a lateral movement to the lower-right. This is a visualization of the motion estimation performed in order to compress an MPEG movie.
Related terms
More often than not, the term motion estimation and the term optical flow are used interchangeably. It is also related in concept to image registration and stereo correspondence. In fact all of these terms refer to the process of finding corresponding points between two images or video frames. The points that correspond to each other in two views (images or frames) of a real scene or object are “usually” the same point in that scene or on that object. Before we do motion estimation, we must define our measurement of correspondence, i.e., the matching metric, which is a measurement of how similar two image points are. There is no right or wrong here; the choice of matching metric is usually related to what the final estimated motion is used for as well as the optimisation strategy in the estimation process.
反思『運動』是『立體』的!投射到『平面』之『圖框』上?焉能完全『適切』耶??
此所以『光流法』探討相對於『觀察者』之『顯運動』 Apparent motion 哩!!
Optical flow or optic flow is the pattern of apparent motion of objects, surfaces, and edges in a visual scene caused by the relative motion between an observer and a scene.[1][2] The concept of optical flow was introduced by the American psychologist James J. Gibson in the 1940s to describe the visual stimulus provided to animals moving through the world.[3] Gibson stressed the importance of optic flow for affordance perception, the ability to discern possibilities for action within the environment. Followers of Gibson and his ecological approach to psychology have further demonstrated the role of the optical flow stimulus for the perception of movement by the observer in the world; perception of the shape, distance and movement of objects in the world; and the control of locomotion.[4]
The term optical flow is also used by roboticists, encompassing related techniques from image processing and control of navigation including motion detection, object segmentation, time-to-contact information, focus of expansion calculations, luminance, motion compensated encoding, and stereo disparity measurement.[5][6]
The optic flow experienced by a rotating observer (in this case a fly). The direction and magnitude of optic flow at each location is represented by the direction and length of each arrow.
Estimation
Sequences of ordered images allow the estimation of motion as either instantaneous image velocities or discrete image displacements.[6] Fleet and Weiss provide a tutorial introduction to gradient based optical flow.[7] John L. Barron, David J. Fleet, and Steven Beauchemin provide a performance analysis of a number of optical flow techniques. It emphasizes the accuracy and density of measurements.[8]
The optical flow methods try to calculate the motion between two image frames which are taken at times
at every voxel position. These methods are called differential since they are based on local Taylor series approximations of the image signal; that is, they use partial derivatives with respect to the spatial and temporal coordinates.
For a 2D+t dimensional case (3D or n-D cases are similar) a voxel at location
with intensity
will have moved by
,
and
between the two image frames, and the following brightness constancy constraint can be given:
-

Assuming the movement to be small, the image constraint at
with Taylor series can be developed to get:
-
H.O.T.
From these equations it follows that:
-

or
-

which results in
-

where
are the
and
components of the velocity or optical flow of
and
,
and
are the derivatives of the image at
in the corresponding directions.
,
and
can be written for the derivatives in the following.
Thus:
-

or
-

This is an equation in two unknowns and cannot be solved as such. This is known as the aperture problem of the optical flow algorithms. To find the optical flow another set of equations is needed, given by some additional constraint. All optical flow methods introduce additional conditions for estimating the actual flow.
就算如是,都還得注意『視野』呀!!
The aperture problem. The grating appears to be moving down and to the right, perpendicular to the orientation of the bars. But it could be moving in many other directions, such as only down, or only to the right. It is impossible to determine unless the ends of the bars become visible in the aperture.
Each neuron in the visual system is sensitive to visual input in a small part of the visual field, as if each neuron is looking at the visual field through a small window or aperture. The motion direction of a contour is ambiguous, because the motion component parallel to the line cannot be inferred based on the visual input. This means that a variety of contours of different orientations moving at different speeds can cause identical responses in a motion sensitive neuron in the visual system.
Individual neurons early in the visual system (V1) respond to motion that occurs locally within their receptive field. Because each local motion-detecting neuron will suffer from the aperture problem, the estimates from many neurons need to be integrated into a global motion estimate. This appears to occur in Area MT/V5 in the human visual cortex.
See MIT example
若是只讀 OpenCV 範例︰
Goal
- In this chapter,
-
- We will understand the concepts of optical flow and its estimation using Lucas-Kanade method.
- We will use functions like cv2.calcOpticalFlowPyrLK() to track feature points in a video.
Optical Flow
Optical flow is the pattern of apparent motion of image objects between two consecutive frames caused by the movemement of object or camera. It is 2D vector field where each vector is a displacement vector showing the movement of points from first frame to second. Consider the image below (Image Courtesy: Wikipedia article on Optical Flow).
It shows a ball moving in 5 consecutive frames. The arrow shows its displacement vector. Optical flow has many applications in areas like :
- Structure from Motion
- Video Compression
- Video Stabilization …
Optical flow works on several assumptions:
- The pixel intensities of an object do not change between consecutive frames.
- Neighbouring pixels have similar motion.
Consider a pixel
in first frame (Check a new dimension, time, is added here. Earlier we were working with images only, so no need of time). It moves by distance
in next frame taken after
time. So since those pixels are the same and intensity does not change, we can say,
Then take taylor series approximation of right-hand side, remove common terms and divide by
to get the following equation:
where:
Above equation is called Optical Flow equation. In it, we can find
and
, they are image gradients. Similarly
is the gradient along time. But
is unknown. We cannot solve this one equation with two unknown variables. So several methods are provided to solve this problem and one of them is Lucas-Kanade.
Lucas-Kanade method
We have seen an assumption before, that all the neighbouring pixels will have similar motion. Lucas-Kanade method takes a 3×3 patch around the point. So all the 9 points have the same motion. We can find
for these 9 points. So now our problem becomes solving 9 equations with two unknown variables which is over-determined. A better solution is obtained with least square fit method. Below is the final solution which is two equation-two unknown problem and solve to get the solution.
( Check similarity of inverse matrix with Harris corner detector. It denotes that corners are better points to be tracked.)
So from user point of view, idea is simple, we give some points to track, we receive the optical flow vectors of those points. But again there are some problems. Until now, we were dealing with small motions. So it fails when there is large motion. So again we go for pyramids. When we go up in the pyramid, small motions are removed and large motions becomes small motions. So applying Lucas-Kanade there, we get optical flow along with the scale.
是否略嫌不足啊??
何不就追本溯源矣☆


KLT is an implementation, in the C programming language, of a feature tracker for the computer vision community. The source code is in the public domain, available for both commercial and non-commerical use.
The tracker is based on the early work of Lucas and Kanade [1], was developed fully by Tomasi and Kanade [2], and was explained clearly in the paper by Shi and Tomasi [3]. Later, Tomasi proposed a slight modification which makes the computation symmetric with respect to the two images — the resulting equation is derived in the unpublished note by myself [4]. Briefly, good features are located by examining the minimum eigenvalue of each 2 by 2 gradient matrix, and features are tracked using a Newton-Raphson method of minimizing the difference between the two windows. Multiresolution tracking allows for relatively large displacements between images. The affine computation that evaluates the consistency of features between non-consecutive frames [3] was implemented by Thorsten Thormaehlen several years after the original code and documentation were written.
Some Matlab interface routines: klt_read_featuretable.m
Note: An alternate Lucas-Kanade implementation can be found in Intel’s OpenCV library. This implementation, described in the note by Bouguet, does a better job of handling features near the image borders, and it is more computationally efficient (approximately 30% on my desktop system). However, it does not contain the affine consistency check. Another alternative is GPU_KLT, which is an implementation of KLT for a graphics processing unit (GPU), which speeds up the run time considerably. A Matlab implementation of a single template tracker is available at Lucas-Kanade 20 Years On. A Java implementation is available here.
References
- [1] Bruce D. Lucas and Takeo Kanade. An Iterative Image Registration Technique with an Application to Stereo Vision. International Joint Conference on Artificial Intelligence, pages 674-679, 1981.
- [2] Carlo Tomasi and Takeo Kanade. Detection and Tracking of Point Features. Carnegie Mellon University Technical Report CMU-CS-91-132, April 1991.
- [3] Jianbo Shi and Carlo Tomasi. Good Features to Track. IEEE Conference on Computer Vision and Pattern Recognition, pages 593-600, 1994.
- [4] Stan Birchfield. Derivation of Kanade-Lucas-Tomasi Tracking Equation. Unpublished, January 1997.